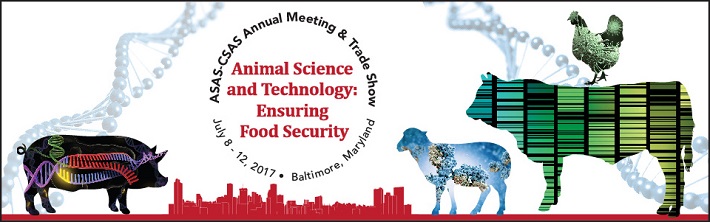
This is a draft schedule. Presentation dates, times and locations may be subject to change.
193
Including Causative Variants into Single Step Genomic BLUP
Including Causative Variants into Single Step Genomic BLUP
Tuesday, July 11, 2017: 12:15 PM
319 (Baltimore Convention Center)
The purpose of this study was determining, by simulation, whether (single-step) GBLUP is useful for genomic analyses when some or all causative Quantitative Trait Nucleotides (QTNs) are known. Simulations included 180,000 animals in 11 generations. Single phenotypes were available for all animals in generations 6-10. Genotypes were available for 24,000 parents in generations 6-10 and 5,000 randomly chosen animals in generation 11. Genotypes included 60,000 SNP (called regular SNP) in 10 chromosomes, with genetic variance fully accounted for by 100 or 1,000 biallelic QTNs. Raw genomic relationship matrices were computed from a) unweighted regular SNP, b) unweighted regular SNP and causative QTN, c) regular SNP with variances from GWA, d) unweighted regular SNP and causative QTN with known variances, e) as before but only using 10% of the largest causative SNPs, and f) using only causative SNPs with known variances. Accuracies for the 11th generation were computed by BLUP and single-step GBLUP. To ensure full rank, raw genomic relationship matrices (GRM) were blended with 1% or 5% of numerator relationship matrix, or 1% of the identity matrix. Inverses of GRM were computed directly or using APY; the APY algorithm exploits limited dimensionality of the GRM for fewer computations and sparse inverse. Rank of GRM with 100 QTN as determined by the number of largest eigenvalues explaining 90% variation in GRM was 8,497 for raw unweighted GRM, increased to 9,553 after blending, decreased to 4,054 with weighted GRM and 10% QTNs included, and was 76 when only causative QTNs were used to create the GRM. The accuracy for the last genotyped generation with BLUP was 0.32. For ssGBLUP with the dense inverse, that accuracy increased to 0.49 with a regular GRM, to 0.53 after adding unweighted QTN markers, to 0.63 when QTN variances were estimated, and to 0.89 when QTN variances were assumed known. When GRM was constructed from QTNs markers only, the accuracy was 0.95 with 5% blending raising to 0.99 with 1% blending. Accuracies assuming 1000 QTNs were generally lower, with a similar trend. Accuracies using the APY inverse were equal or higher than those with a regular inverse. The rank of weighted GRM is between the rank of unweighted GRM and that computed with QTNs only. Single-step GBLUP can account for causative SNP with nearly optimum accuracy when variances of causative QTN are known.