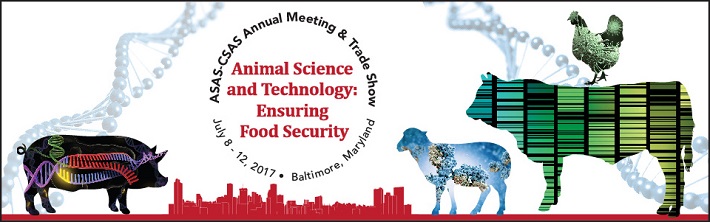
This is a draft schedule. Presentation dates, times and locations may be subject to change.
162
Comparison of Genomic-Polygenic Evaluations Using Random Regression Models with Legendre Polynomials and Splines for Milk Yield and Fat Percentage in Thai Multibreed Dairy Cattle
Comparison of Genomic-Polygenic Evaluations Using Random Regression Models with Legendre Polynomials and Splines for Milk Yield and Fat Percentage in Thai Multibreed Dairy Cattle
Monday, July 10, 2017
Exhibit Hall (Baltimore Convention Center)
The objective of this research was to compare random regression models with third-order Legendre polynomials (RRLP) and linear splines of four knots (RRSP4) for milk yield (MY) and fat percentage (FP) genomic-polygenic evaluations in a Thai multibreed dairy cattle population. Models were compared using estimates of variance components, genetic parameters, model-fit criteria, accuracy of genomic EBV, and animal rankings. The dataset contained pedigree and first-lactation monthly test-day records (69,029 for MY; 29,878 for FP) of 7,206 cows from 761 farms. Genotypic information included 74,144 actual and imputed SNP markers from 2,661 animals. Variance components and genetic parameters for MY and FP were estimated using REML procedures. The single-step random regression genomic-polygenic models included contemporary group (herd-year-season), calving age, heterozygosity, and population lactation curve regression coefficients as fixed effects. Random effects were animal additive genetic random regression coefficients, permanent environment random regression coefficients, and residual. The effects of population lactation curve, animal additive genetic, and permanent environment were fitted using regression coefficients of third-order Legendre polynomials for RRLP and splines of four knots for RRSP4. Results showed estimates of 305-day additive genetic variances and heritabilities for MY and FP to be higher for RRLP (additive genetic variances: 279,893.2 kg2 for MY, 0.10 %2 for FP; heritabilities: 0.27 for MY, 0.16 for FP) than for RRSP4 (additive genetic: 260,178.1 kg2 for MY, 0.08 %2 for FP; heritabilities: 0.19 for MY, 0.11 for FP). Similarly, RRLP yielded a better model-fit and higher prediction accuracies than RRSP4. The RRLP had lower values of -2 log-likelihood (-2logL; 293,813), Akaike’s information criteria (AIC; 293,855) and Bayesian information criteria (BIC; 293,915) as well as higher prediction accuracies (49.25% for MY; 38.63% for FP) than RRSP4 (-2logL = 362,738; AIC = 362,888; BIC = 363,101; prediction accuracies: 47.23% for MY, 37.76% for FP). However, rank correlations between genomic EBV from RRLP and RRSP4 were high for MY (0.94) and FP (0.90). Results indicated that genomic EBV from RRLP should be preferred to those from RRSP4 to help increase selection responses for MY and FP in the Thai multibreed dairy cattle population.