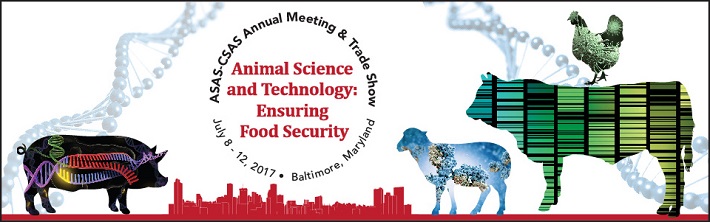
This is a draft schedule. Presentation dates, times and locations may be subject to change.
211
Joint Genome Wide Association Analysis of Continuous and Discrete Traits
Joint Genome Wide Association Analysis of Continuous and Discrete Traits
Tuesday, July 11, 2017
Exhibit Hall (Baltimore Convention Center)
Genome wide association studies (GWAS) are becoming a standard tool for the genetic dissection of complex traits and for the estimation of genomically enhanced breeding values. Although linear regression models for implementation of association studies were used to analyze continuous and discrete responses, their implementation was always in a univariate context. Arguably, the joint analysis of continuous and discrete traits in GWAS will be advantageous because of a better use of available information and the existing correlation structure among traits. However, a joint association analysis for multiple continuous and discrete traits presents several theoretical and implementation complexities. In presence of binary traits in the analysis, the residual (co)variance matrix is not complete random due to the fixation of some diagonal elements which complicates substantially the sampling process. The residual updating algorithm often used to solve the system of equations in GWAS analyses requires some changes to accommodate the changing liabilities of discrete responses each round of the sampling process. Missing traits, more frequently for discrete responses, add another layer of implementation complexity. In order to investigate the advantages of a joint analysis of continuous and discrete responses, a real data based simulation was carried out. Two continuous, one binary and one multinomial trait with heritability of 0.3, 0.4, 0.1, and 0.1, respectively and varying covariance structure were simulated. Traits were generated following a linear model that included three systematic effects, 100 QTLs and error terms. The data consisted of 1,365 animals genotyped for 41,694 SNPs. A random missing rate of 10% was assumed for the discrete responses. Each of the four traits was analyzed separately using either a linear (for continuous responses) or a threshold (for discrete responses) model. The four traits were also analyzed jointly using a linear-threshold model. For the univariate and multivariate analyses, a BayesA-like approach was implemented. For all analyses a 5 fold cross validation was carried out. Using the univariate analyses, the accuracy (correlation between true and estimated breeding values) was 0.48, 0.62, 0.31 and 0.28 for the continuous, binary and categorical traits, respectively. Multivariate analyses resulted in a 4 to 17% increase in the accuracy of discrete traits dependent of the covariance structure. However, for the continuous responses the accuracy increased only when the correlations between traits exceeded 0.1. For moderate to high correlations, the accuracy of all continuous traits increased by 2 to 6%. Joint analyses resulted in substantial increase in computational costs.