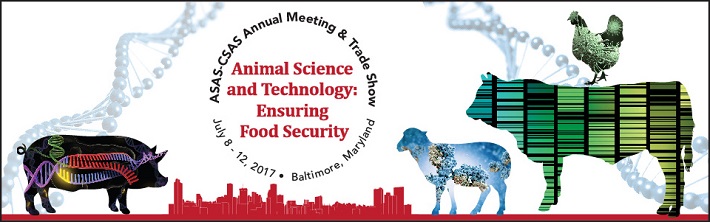
This is a draft schedule. Presentation dates, times and locations may be subject to change.
748
Mining Farm- and Animal-Level Data to Optimize Beef Cattle Production
Mining Farm- and Animal-Level Data to Optimize Beef Cattle Production
Wednesday, July 12, 2017: 11:35 AM
315 (Baltimore Convention Center)
Data mining is defined as the practice of examining large databases in order to generate useful information from discovered patterns. Although the term “large databases” is relative and context dependent, the concept of analyzing datasets to generate predictive models is a key part of data mining. Data mining in livestock systems include both the animal- and farm-level components. At the animal level, measurements such as feed intake, gain, diet and milk composition, health recordings, behavior, etc., can be collected. In recent years, sensor systems have been developed to aid in cattle management. For instance, sensors are able to measure rumination time, activity, lameness, health indices, and grazing time among other variables. In addition, image analysis has become an important tool to measure body characteristics such as carcass composition in beef cattle and backfat thickness in dairy cows. Farm-level data reflect a larger picture of livestock systems where management and economic factors play an important role. Those factors can include cattle sales price, feed price, transportation costs, medication, herd size, nutritional strategy, weather condition, etc. To illustrate farm-level data analytics, we will present two different datasets. The first one refers to data on Brazilian beef cattle from two sources: JBS S.A. (81,053 farms) and DSM Produtos Nutricionais (22,223 farms). The combined dataset comprised 7,248 farms and 1,571,023 carcasses slaughtered in the past three years (2014-2016). In this first example, we investigated the effect of different nutritional supplements, adoption of frequent technical consulting, season, animal category, and other variables on carcass quality, body weight and age at slaughter. The second dataset is from CMA, a cattle feeding company in Brazil, consisting of 777 pens and 62,992 cattle slaughtered in the past 3 years (2014, 2015 and 2016). Seventeen economic and management variables such as breed, days on feed, diet cost, transportation, medication, season and others were used to predict profitability. The models were developed to create a decision-making tool for earlier prediction of negative profits in feedlot systems. Interest on data mining in livestock systems has markedly increased and reflects the necessity to uncover hidden patterns present in large datasets and transform them into useful information.