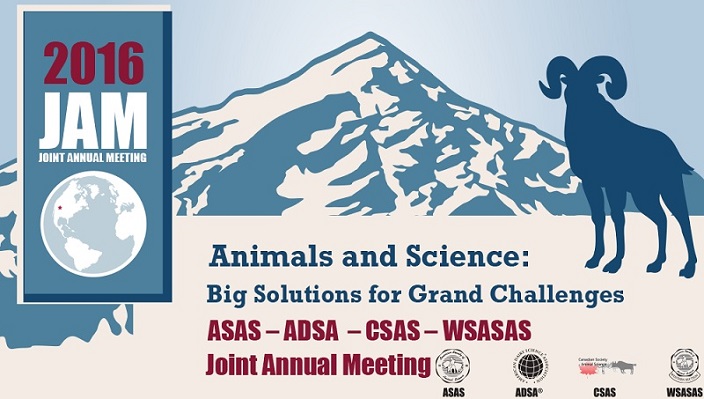
Some abstracts do not have video files because ASAS was denied recording rights.
793
Statistical models and tools for Integration of omics data to uncover the genetic control of pork quality and growth traits
Next generation sequencing is drastically changing the way we study the interactions between genomes, transcriptomes and phenomes. The next generation of animal genomics datasets should be matched by the next generation of analysis models. Those models should be: flexible and adaptable to complex designs pervasive in animal genomics, statistically sound to test hypotheses and estimate parameters that are important for animal genomic research, computationally efficient and able to accommodate massive multilayer datasets that are typical of animal genome research. The goals of this presentation are to 1) propose models for genome-wide association (GWA) that can be used for expression QTL (eQTL) and phenotypic QTL (pQTL) mapping and to show their properties using simulations and real data analyses. As part of the analysis, we specify significance thresholds to control type I error rate, we compute confidence intervals of QTL positions using cross-validation and we demonstrate under simulation that intervals have the intended coverage. Finally, we derive a meta-analysis procedure to integrate results of several independent GWA. We use plasmode simulations to show that our proposed method has a series of desirable properties for implementation of high-throughput association analyses: a) computational cost scales linearly with the number of markers allowing rapid GWA with massive datasets, b) Genetic substructure and relationships among animals are fully accounted for, and c) Type I error rates are controlled at nominal levels. Finally, we present graphical and descriptive summary tools to represent results of massively parallel GWA analyses. To illustrate the usefulness and computational efficiency of proposed models we present results from several projects: 1) GWA analysis of over 50 pork quality, carcass composition and growth traits for almost 1000 DurocxPietrain F2 pigs, 2) GWA of over 40000 gene expression phenotypes from backfat and longissimus muscle tissues of 176 individuals of the same population, and 3) meta-analysis of five meat quality traits across three divergent pig populations. We show that using our proposed methods we can map eQTL overlapping pQTL and confirm their joint association. More importantly, we demonstrate that such mapping can be performed and represented in an unsupervised way (with minimal human input) by integrating genome-wide expression and genotyping, as well as high throughput phenotyping data through proper data analysis models and tools.
Keywords: eQTL, GWA, genetical genomics