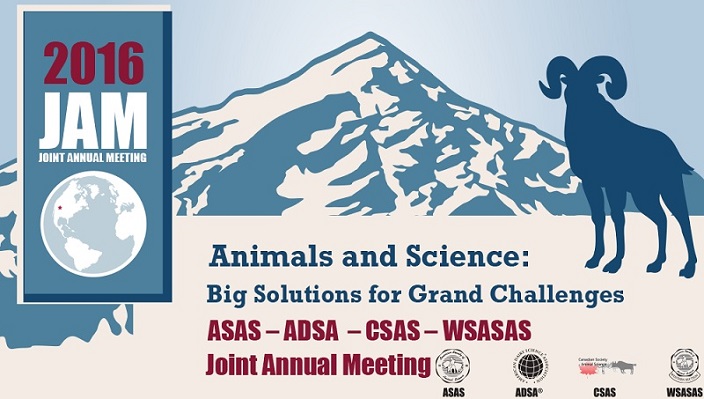
Some abstracts do not have video files because ASAS was denied recording rights.
784
Multi-omics data resources and use in genetic improvement of cattle growth and health
Interactions of growth and health influence the progression of cattle to maturity. Growth and carcass traits typically have moderate to high heritability (h2: 0.2-0.5), whereas pathogen-disease related health traits tend to have low heritability (h2 ≤ 0.2). Genetic improvement in beef cattle involves selection with EPD predicted from multi-trait, and often multi-breed, models that incorporate genomic data derived from SNP-chips. Available genotyping platforms have genome-wide distance gaps of 49.4 kb (BovineSNP50) and 3 kb (BovineSNPHD). Genetic prediction accuracy can be improved with use of genomic data; however, process varies greatly depending on trait heritability and quality-quantity of data. Since most economically relevant traits are polygenic and single-SNP within a positional-candidate gene explains limited variation, there is great interest in discovery and use large numbers of causal-mutation SNP in genetic prediction. These SNP will likely be located within nodes and hubs of gene networks and can be added to the current chips to improve their effect. Various “omics” tools evaluating transcriptome, proteome, and metabolome assist with discovery of coding and tag SNP. Differentially expressed targets from the latter two tools reveal gene-products closely associated with an animal’s physiologic-phenotype, which can correlate with gene expression levels observed in RNA. Concordant results and enrichment analyses from these tools yield confidence to analyze target sequence, either DNA or RNA, to discover functional SNP. However, transcript splicing, peptide processing, and post-translational modifications complicate comparing results from the various approaches. The animal’s epigenome and microbiome also influence phenotype. To identify functional SNP, RNA must be harvested from tissues of animal models designed to be informative for a trait collected from large breeding populations. Case versus control or comparisons of cattle from the tails of the quantitative trait distribution curves have proven useful strategies in several multi-omics growth and health studies of which pulmonary arterial pressure (PAP, h2: 0.2-0.4), an indicator of hypoxia-induced pulmonary hypertension, is an example. Here, cardiopulmonary tissues were collected from high and low PAP Angus steers (n = 10/group) with sire-pedigree diversity to growing seedstock bulls. Transcript abundance was assessed and differential expression results were obtained and are being merged with QTL and whole-genome sequence information to discover SNP-genotypes for incorporation into a PAP EPD, a tool for selecting cattle for high elevation tolerance. Therefore, strategic application of multi-omic resources to discover functional SNP and obtain population-level genotype-data provides opportunity to enhance EPD accuracy.
Keywords: Cattle, Growth, Health, Omics