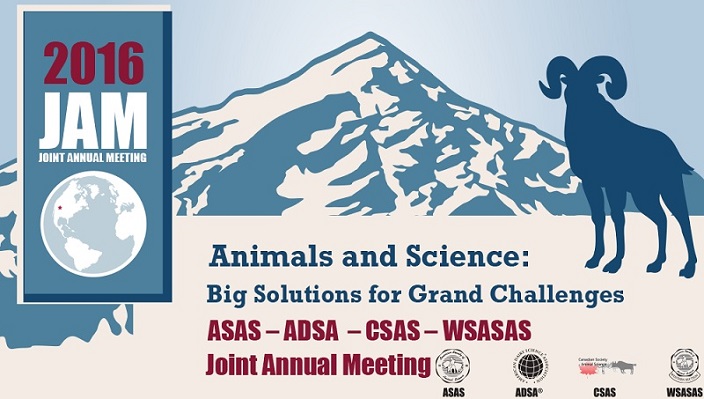
Some abstracts do not have video files because ASAS was denied recording rights.
1296
Evaluation of multilevel mixed effect models
Simple mixed effect models have been extensively used in animal science literature. However, in some instances biological relationships require that models account for deviations of individual animals from that of the population. Furthermore, some animals might share similar genetic background because they are closely related (e.g., pig littermates) thus specification of animal within litter relationship (i.e., nested random effects) is necessary in order to model the hierarchical data structure. In some cases measurements taken on the same individual may not be independent (e.g., weekly BW measurements). This will result in models with heteroskedastic and serial correlated errors, which need to be evaluated and the errors minimized. Recent developments in statistical theory and computational power allow for specification of multilevel mixed effect models, especially nonlinear models. To demonstrate implementation of such models, an example is provided using data collected from an experiment with 40 pigs of 3 sexes originating from 17 litters and their BW measured weekly or every 2 wk up to 1,007 d. A multilevel mixed effects model was used within a growth function because it allows for estimation of all growth profiles simultaneously, and different sources of variation. Furthermore, variance in-homogeneity and within-animal correlation were introduced to the growth function. In the basic model, the variance was assumed to equal to identity matrix, i.e., the within-animal errors are independent, identical and random vectors. The basic model fit suggested that the within-animal variability increased with increasing BW and auto-correlation was also present. The variance-covariance matrix was then relaxed and decomposed into variance structure component and a correlation structure component that allows specification of model variance heterogeneity and serial correlation. Variance of the within-animal errors was modeled using a variance function, which when implemented reduced Bayesian Information Criteria (BIC) values to 8950 compared to 9861 for the basic model but did not remove the strong auto-correlation in the residuals. A continuous time autoregressive process of first order was applied to the within-animal errors because it deals with unequally spaced observations. This further reduced BIC to 7146 due to removal of the serial correlated errors and thus inclusion of a continuous auto regressive process of first order is recommended when modeling frequently sampled growth data.
Keywords: multilevel mixed effect model, variance structure, autocorrelation