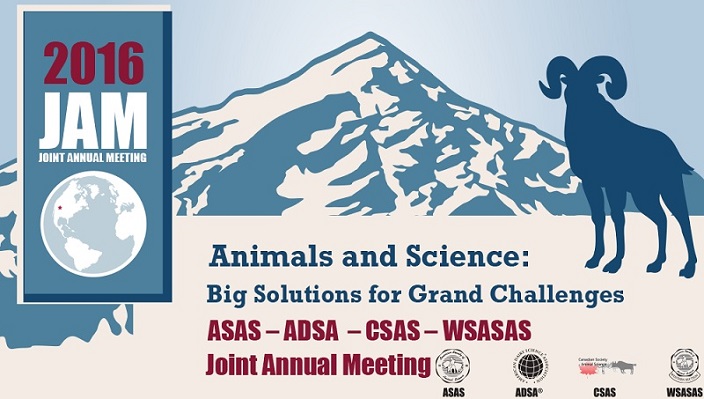
Some abstracts do not have video files because ASAS was denied recording rights.
300
SNP filtering using Fst and implications for Genome wide association and phenotype prediction
Although genome-wide association studies (GWAS) detect single nucleotide polymorphism (SNP)-trait association, these SNPs explain only a small fraction of the variation. Genomic selection (GS), through the use of all available genetic information, tends to have a better dissection of the phenotypic variation, but its performance is still far from optimum. GWAS and GS are affected by lack of power is due to small sample size, large number of highly correlated markers, and the moderate to small effects of most QTLs. This situation could get even more complex with the continuous increase in marker density. Methods that internally try to prioritize SNPs (i.e. BayesB) tend to provide a certain relief when low to medium density panels are used but their advantages degrade with the increase of the number of markers. Thus, it is becoming a necessity to either perform a SNP filtering before conducting the association analysis or to enlist additional external sources of information. Knowledge of genetic diversity based on evolutionary forces is beneficial for tracking loci influenced by selection. FST, as measure of allele frequency variation among populations, provides a tool to reveal genomic regions under selection pressure. In order to evaluate its usefulness as an external source of information in association studies, a simulation was carried out. A trait with heritability of 0.4 was simulated and three sub-populations were created based on the empirical phenotypic distribution (< 5% quantile; > 95% quantile and between 5 and 95% quantiles). Marker data was simulated to mimic 600K and 1 million SNP panels. Genetic complexity of the trait was modeled by the number of QTLs, their distribution, and magnitude of their effects. Using different empirical cut off values for FST, most QTLs were correctly detected using as little as 0.8% of SNP markers in the panels. Furthermore, the genomic similarity base on the selected SNPs was very high (>0.80) for individuals with similar genetic and phenotypic values even that they have limited to no blood relationship. These results indicate that filtering SNPs using FSTcould be beneficial to GWAS and GS by focusing on genome regions under selection pressure. This could be relevant with the availability of next-generation-sequencing data. High functional genomic similarity based on selected markers indicates similarity in SNP signatures, regardless of blood relationships, and translates into high phenotypic correlation that could be used in decision making.
Keywords: GWAS, FST, genetic and phenotypic prediction