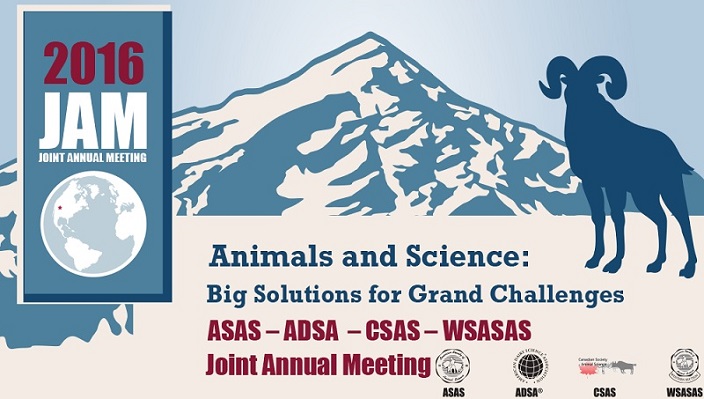
Some abstracts do not have video files because ASAS was denied recording rights.
291
APY inverse of genomic relationship matrix – theory, analyses and questions
Genomic relationship matrix (GRM) can be inverted by Algorithm for Proven and Young (APY) based on recursion on a random subset of animals. While a regular inverse has a cubic cost, the cost of the APY inverse can be close to linear, allowing inexpensive computations with millions of genotyped animals. Theory proposed for APY assumes that optimal size of the subset (maximizing accuracy of genomic predictions) is due to a limited rank of GRM, which is a function of independent chromosome segments (Me) and subsequently of effective populations size (Ne). Simulation studies have shown that 1) the dimensionality is almost a linear function of Ne but for large Ne can be depressed by limited number of genotyped animals and SNP markers, 2) accuracy of predictions with APY inverse is higher than with a regular inverse, 3) the distribution of independent chromosome segments is skewed. Tests using commercial data sets confirmed results by simulation. Comparisons of eigenvalue plots between simulated and commercial populations indicated an effective population size of 157 for Holsteins, 115 for Angus, 107 for Jerseys, 41 for broiler chicken and 30 for pigs. Experiences with the APY inverse raise a few questions. Can the rank provide information on the minimum SNP chip size that eliminates the polygenic component (or missing heritability)? Is the rank of GRM for a large two-breed population twice that of a single population? In simulation studies where QTL are on SNP markers, the best correlation of a simulated QTL effect is not with the actual SNP effect but with an average of adjacent SNPs. Is the optimum number (window size) of adjacent SNP a function of Ne and dictates the maximum resolution in GWAS? With all causative SNP are identified and their variances known, and appropriate weighted GRM (with APY inverse applicable) has the rank of the number of causative SNPs. Is the rank of weighted GRM with incomplete identification of QTLs (e.g., via GWAS or BayesB) smaller than that of a regular GRM? The APY inverse solves the problem of large-scale genomic computations and provides new insight into the genomic information.
Keywords: Genomic selection, single-step GBLUP, APY inversion