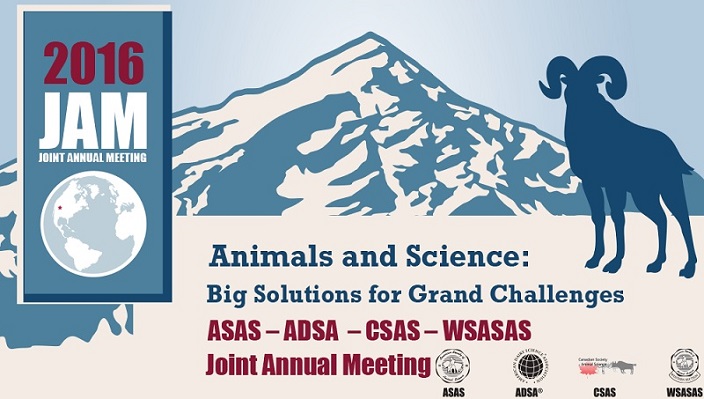
Some abstracts do not have video files because ASAS was denied recording rights.
293
Accounting for discovery bias in genomic prediction
Our objective was to evaluate an approach to mitigating discovery bias in genomic prediction. Accuracy may be improved by placing greater emphasis on regions of the genome expected to be more influential on a trait. Methods emphasizing regions result in a phenomenon known as “discovery bias” if information used to determine influential regions is also used to predict genetic merit. Discovery bias causes genomic predictions to appear to be more accurate than they actually are. Generally, EBV of a population are conditional on as much information as possible and individual EBV are each conditional on exactly the same information. An analysis of simulated data (105 replicates) was conducted to test whether discovery bias could be reduced and true accuracy of prediction could be improved by relaxing the constraint that all EBV are conditional on the same information. In the default analysis, molecular breeding values (MBV) were computed from 2487 random SNP effects whose variances were estimated by REML. The 2600 phenotypes were simulated for non-parent animals only, which were progeny of 107 sires with number of paternal half-sibs per group ranging from one to 107. Corrected MBV (CMBV) were computed for each paternal half sib group by repeating the REML analysis on a data set that excluded records within that paternal half-sib group in an attempt to reduce discovery bias. True accuracy (correlation of MBV or CMBV with simulated breeding value) was lower for CMBV than for MBV. To recover the lost information without reintroducing discovery bias, a two-trait pedigree-based post-analysis was performed in which all 2600 phenotypes were fit as the first trait and the MBV (CMBV) were fit as the second trait. The solutions for the first trait are referred to as EBV and CEBV, respectively. True accuracy was greater for EBV than for MBV, suggesting the pedigree captured some genetic variance not accounted for by SNP. True accuracy was greater for CEBV than for EBV. Model derived accuracies were computed from prediction error variances of animals or functions of marker effects in the respective models. All model derived accuracies were greater than the corresponding true accuracies, indicating that discovery bias was present. Model derived accuracy was closer to true accuracy for CEBV than for EBV, indicating that the proposed correction was successful in reducing discovery bias, although it did not completely remove it. USDA is an equal opportunity employer.
Keywords:
accuracy, discovery bias, genomic prediction