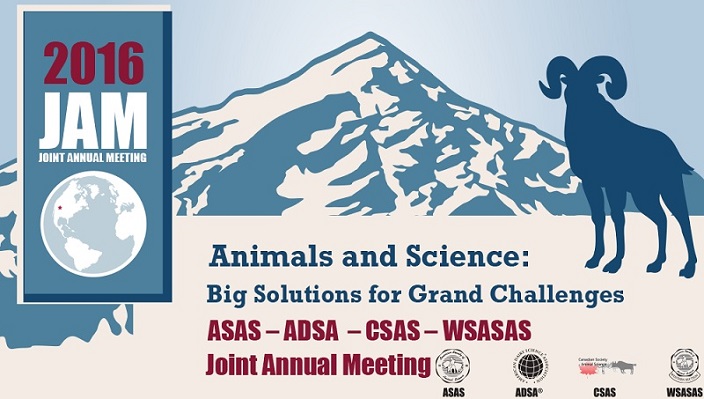
Some abstracts do not have video files because ASAS was denied recording rights.
297
High density marker panels, SNPs prioritizing and accuracy of genomic selection
High density marker panels, SNPs prioritizing and accuracy of genomic selection
Wednesday, July 20, 2016: 11:15 AM
Grand Ballroom I (Salt Palace Convention Center)
Abstract Text: Availability of high density (HD) SNP marker panels, genome wide variants and even sequence data create an unprecedented opportunity of dissect the genetic basis of complex traits and to enhance selection in livestock and plant species. The disproportional increase in the number of parameters in the genetic association model compared to the number of phenotypes has led to further deterioration in the statistical power, and increase in co-linearity and false positive rates. HD panels do not improve the accuracy of GS in any significant manner and could even lead to reduction in accuracy using both regression and variance component methods. As a result, HD panels at best they did not improve significantly the accuracy of genomic selection and at worst they led to a reduction in accuracy. This is true for both regression and variance component approaches. To remedy this situation, either some form of SNP filtering or external information is needed. Current methods for prioritizing SNP markers (i.e. BayesB, BayesC) are sensitive to the increased co-linearity in HD panels which could limit their performance. In this study, the usefulness of Fst, a measure of allele frequency variation among populations, as an external source of information in genomic selection was evaluated. A simulation was carried out for a trait with heritability of 0.4. Data was divided into three subpopulations based on trait distribution (top 5%, bottom 5% and in between). Marker data was simulated to mimic 770K SNP marker panel. A ten chromosome genome with 200K SNPs was simulated. Several scenarios with varying number of QTLs and their associated effects were simulated. Fst empirical cutoff values of 0.004, 0.008, 0.01, and 0.02 were used to prioritize markers resulting in 4579, 2288, 1745, and 650 selected SNPs, respectively. Using all 200K markers and no filtering, the accuracy of genomic prediction (correlation between true and predicted breeding values) was 0.48. When SNPs were pre-selected based on Fst, accuracy was 0.41, 0.48, 0.49, and 0.53 for Fst cutoff values of 0.004, 0.008, 0.01, and 0.02, respectively. It is clear that the accuracy obtained using all SNPs can be easily achieved using only 0.5 to 1% of all markers. These results indicated that SNP filtering using already available external information could increase the accuracy of genomic selection. This is especially important as next generation sequencing technology is becoming more affordable and accessible to animal and plant applications.
Keywords: SNP prioritizing, genomic selection, high density