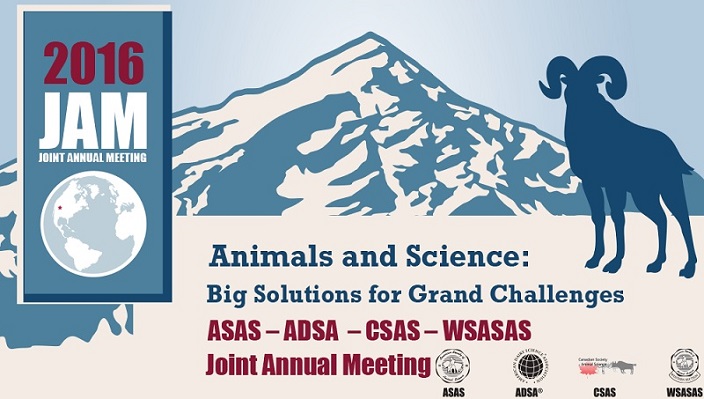
Some abstracts do not have video files because ASAS was denied recording rights.
34
Opportunities and challenges for herd health and reproduction with robotic milking
There has been a rapid increase in the number of herds with automatic milking systems (AMS). This technology is a well-established means to harvest milk from cows. Robotic milking offers potential advantages in labor per cow, increased milking frequency, and integration of sensors and data collection that assist with estrus detection and might help with detection of health problems or lameness. Activity monitoring (AM) systems (in AMS or parlor-milked freestall barns) have been shown to produce, on average, comparable herd pregnancy rates to alternative approaches to reproductive management. However, AM requires supplemental interventions for timely AI for approximately 20% of inseminations. AMS provide streams of a variety of data on activity, milking frequency and timing, quarter-level milk yield and conductivity and the daily cow-level variation in these metrics. The systematic collection of these data offers the promise of detection of some health problems earlier and with less variation. However, selection of valid, actionable indicators of health from AMS data remains a work in progress, and a balancing act of adequate detection and false alerts. As with conventional systems, the associations of AMS with cow health, welfare, and performance are confounded by their human managers. There is little data on measures of health with AMS. More research is needed on the predictive value of indicators of mastitis from AMS. Research on approaches to and outcomes of treatment of mastitis with AMS-based detection is lacking. Reports indicate a similar prevalence of lameness to non-AMS freestall barns, with similar stall- and bedding-related factors associated with lameness, injuries, and lying time. Processing of multiple data inputs, mostly related to cow activity and milking performance, has been shown to have reasonable accuracy for detection of lameness, which if successfully implemented would likely be earlier and more thorough. Preliminary data indicate a higher prevalence of ketosis in herds with AMS. Feeding behavior is generally driven by feed delivery as in non-AMS barns, such that feeding space requirements are similar. Timely detection of metabolic disease such as ketosis may be aided but not replaced by AMS-derived data, and detection of reproductive disease still requires daily observation of the cows by skilled people; pen design and cow handling equipment are needed to facilitate interactions with the cows.
Keywords: health, disease detection, precision technology