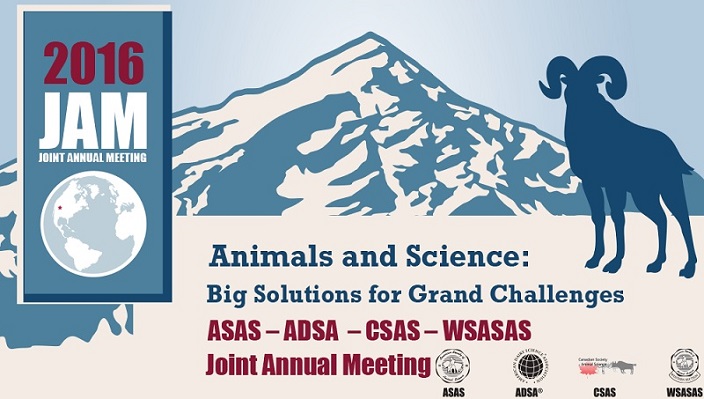
Some abstracts do not have video files because ASAS was denied recording rights.
415
Improving genomic selection across breeds and across generations with functional annotation
Identification of causal mutations which affect complex traits in livestock (including production, health and fertility) could accelerate genetic gains for these traits by improving the accuracy of genomic estimated breeding values, particularly across breeds and with greater persistency of accuracy across time. Identification of these causal mutations could also reveal facets of the biology underlying such traits. A significant proportion of the genomic variation in cattle, for Bos taurus breeds at least, has been identified. The 1000 bull genomes project now includes whole genome sequences from 1682 cattle of 55 breeds, from which 67.3 million variants (64.8 million SNP, 2.5 million indel) have been identified. The challenge is now to determine which subset of these variants affect complex traits. This challenge is magnified by the fact that the size of effects of the causal mutations are likely to be small, given the large number of mutations typically affecting complex traits. We propose that an approach that includes 1) a multi-breed reference population (necessary to break down the extensive linkage disequilibrium that exists within many livestock breeds) 2) intermediate phenotypes, such as gene expression and protein abundance, where mutation effect is much larger than on the complex trait phenotype 3) genome annotation information, to identify which classes of variants are more likely to affect complex traits, and 4) a genomic prediction algorithm that uses all this information simultaneously, will lead to identification of causal mutations on a genome wide scale. Several examples identifying potential causal mutations affecting milk composition from dairy cattle are given. The results highlight the need for better annotation of the bovine genome – many of the most significant mutations are in poorly annotated genomic regions, likely regions regulating gene expression. The functional annotation of animal genomes (FAANG) consortium will greatly improve this situation.
Keywords: genomic selection, functional annotation