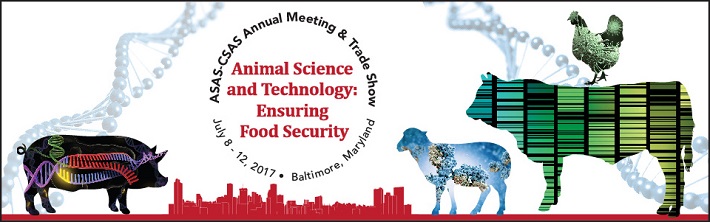
This is a draft schedule. Presentation dates, times and locations may be subject to change.
187
Analysis of Misclassified Categorical Responses
Analysis of Misclassified Categorical Responses
Tuesday, July 11, 2017: 10:30 AM
319 (Baltimore Convention Center)
Misclassification in categorical outcome variables is a common and difficult issue that results in biased inference. Several traits of economic and welfare importance in animal improvement programs are discrete in nature. The recording of these traits is prone to misclassification for several reasons, including subjectivity of measurement, improper recordkeeping, or simply changes in the definition of the traits over time. Although several methods have been proposed to deal with misclassification in binary outcome variables, to the best of our knowledge there is no methodology that has been applied to the analysis of multinomial responses subject to misclassification. In this study, we proposed a method for analysis of misclassified ordered categorical responses through the extension of our previous work on dealing with noisy binary data. The proposed method identifies potentially misclassified observations, adjusts their status and conducts the analysis with the corrected data. To evaluate the effectiveness of the proposed method, a simulation study was carried out. Two data sets of 10K and 1.5K records were simulated. The latter was simulated following the structure of an existing beef cattle calving ease data set. For both data sets a discrete response with three classes (70%, 20%, and 10% incidence rate) and a heritability of 0.1 was simulated. A misclassification rate of 5% was randomly introduced to the data set by switching the true response to the two alternative outcomes with equal probability. True and misclassified data sets were analyzed by two approaches: a classical threshold model that does not account for misclassification (M1) and by our proposed model that contemplates potential misclassification (M2). Each simulation scenario was replicated 10 times. For all scenarios, when the true data sets were analyzed the true parameters were estimated without bias, although the estimates using the small data set had large posterior standard deviation, as expected. When the misclassified data was analyzed with M1, a 20.5% and 11.8% bias was observed in the estimation of the heritability for the large and small data sets, respectively. Using M2, bias was removed. In fact, estimates of heritability were almost identical to those obtained using the real data (0.106 vs. 0.106 and 0.097 vs 0.098 for 10K and 1.5K data sets, respectively). Furthermore, the proposed method was able to detect true misclassified records with high probability. These results clearly indicate the effectiveness of the proposed method in reducing bias in the analysis of discrete data subject to misclassification.