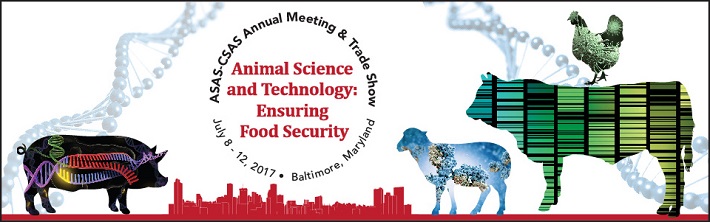
This is a draft schedule. Presentation dates, times and locations may be subject to change.
186
A Hybrid of Prioritized SNP and Polygenetic Effect Method for Implementation of Genomic Selection
A Hybrid of Prioritized SNP and Polygenetic Effect Method for Implementation of Genomic Selection
Tuesday, July 11, 2017: 10:15 AM
319 (Baltimore Convention Center)
Genomic selection (GS) is rapidly becoming the method of choice for genetic evaluation. GS is implemented using either a linear regression or mixed effect models. The continuous enhancement of the density of marker panels and the number of genotyped animals are creating major theoretical and implementation problems to both approaches. Furthermore, little to no improvement in accuracy of GS was achieved using this high density (HD) genomic data. This lack of improvement in accuracy is not due to the lack of informativeness of the HD data, rather because of the inability of existing methods to harness additional information. This is the case due to lack of statistical power using linear regression models and the limited improvement of the genomic relationship matrix (G) for the mixed linear model approach. The later suffers additionally from the increase in the number of genotyped animals which complicates substantially the inversion of G. Thus, it is reasonable to assume both problems could be solved or at least attenuated if SNP markers are prioritized not based on their estimates effects (i.e. BayesB) but rather on some other criteria that are not affected by the limited statistical power. Only the “prioritized” SNPs will be included in the association model and a polygenic term will be added to account for the remainder of the genetic merit. This hybrid method is a compromise between both existing approaches. In this study, fixation index (FST) scores were used as criteria to prioritize SNPs. To evaluate hybrid method, a simulation was carried out for 15k genotyped animal with 50k SNP panel. The heritability of a trait (0.4) was totally explained by 200 QTLs which 12%, 33%, and 66% of them explain at least 1%, 0.5% and 0.3% of the total genetic variance (GV), respectively. No polygenic component was simulated. By using the 97.5 quantile of the distribution of FST scores to prioritize SNPs, the proposed method resulted is a more accurate estimate of heritability (0.36) compared to BayesB (0.32). Although both methods have similar estimates of the portion of GV explained by the genomic information, the proposed method induces a reduction in the residual variance due to a non-zero estimate of the polygenic variance (0.10). Accuracy was similar between hybrid and BayesB methods (0.48 vs. 0.49). However, the new approach does not require the inversion of G and is able to handle genotyped and non-genotyped animal with limited increase in computational cost.