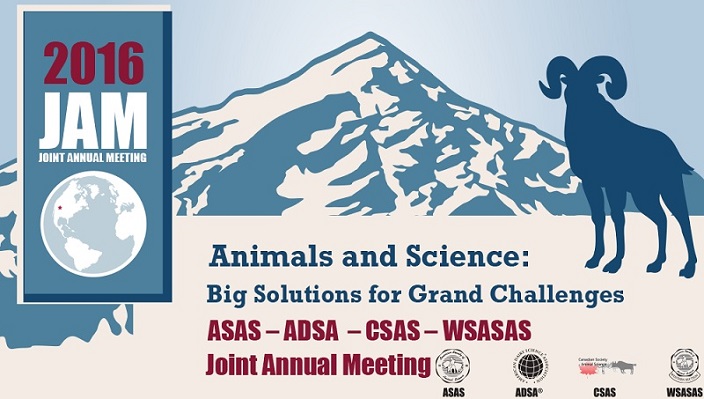
Some abstracts do not have video files because ASAS was denied recording rights.
305
Heteroskedastic extensions for genome-wide association studies
Bayesian multiple regression models based on genomic marker information are commonly used for genomic prediction and selection and are being increasingly utilized in genome-wide association (GWA) analyses to search for genomic regions associated with economical important traits in agriculture. These models jointly fit all markers, thereby circumventing the limitations of “one-marker-at-a-time” of traditional GWA inference. We have recently validated and tested extensions of genomic prediction models to account for residual heteroskedasticity, which is prevalent in livestock field data. Our objective was to evaluate the impact of not accounting for potential residual heteroskedasticity in GWA inference. Using simulated data scenarios that reflected a gradient of increasing residual heteroskedasticity, we fitted homoscedastic and heteroskedastic error versions of hierarchical Bayesian genomic prediction models assuming either normal (RR-BLUP) or heavy-tailed (BayesA) prior specifications on the effects of genomic markers. For each marker, we then constructed a posterior z-score using prediction error variance of the estimated marker effect to detect associations between genomic regions and phenotypes of interest. Under conditions of extreme heterogeneity of residual variances, heteroskedastic models showed an increase in power of up to 10 percentage points for GWA discovery with little impact on false positive rate (i.e. change of 0 to 3 percentage points), compared to the homoscedastic model counterparts. Further, when heteroskedasticity was high, the absolute magnitude of the estimated signal for the most prominent QTL expressed as a posterior z-score was enhanced by 20% and 34% for heteroskedastic RR-BLUP and BayesA, respectively. The inferential advantages of heteroskedastic models over homoscedastic ones were particularly apparent under a BayesA specification. A data application involving three quantitative carcass and meat quality traits from a swine resource population representing high, mild and low levels of heteroskedasticity yielded proportionally enhanced differential detection signal for the heteroskedastic models relative to the homoscedastic ones, consistent with results from the simulation study. In conclusion, accounting for residual heteroskedasticity can be expected to enhance power in the identification of important genomic regions for traits of interest.
Keywords: genome-wide association, residual heteroskedasticity, genomic prediction model.