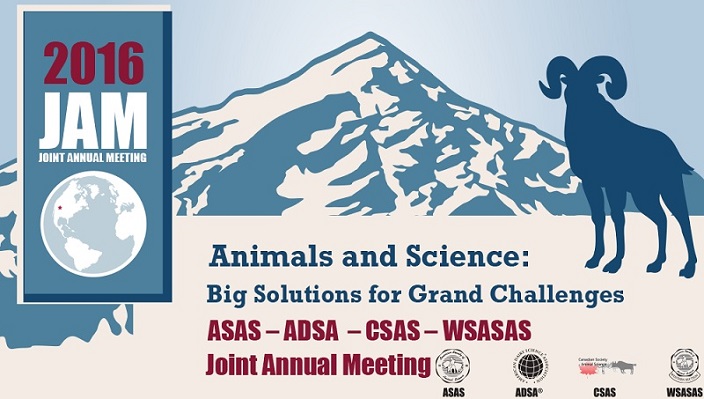
Some abstracts do not have video files because ASAS was denied recording rights.
1407
Automation of statistical procedures to screen raw data and construct feed composition databases
Automation of statistical procedures to screen raw data and construct feed composition databases
Wednesday, July 20, 2016: 2:45 PM
155 E (Salt Palace Convention Center)
Abstract Text: Millions of feed composition records have been generated from feed testing laboratories annually, providing high-valued assets that could be leveraged to benefit the animal nutrition community. Unfortunately, managing, handling, and processing feed composition data that originate from multiple resources are challenging, due in part to inconsistencies of how data are reported and the time needed to develop databases. Methods that consolidate and utilize these data are needed to develop accurate and precise feed composition databases. The objectives of this project were to: 1) develop automated statistical procedures to screen for outliers of feed composition data obtained from multiple resources; and 2) evaluate the efficiency of these procedures on classifying feedstuffs. A published statistical procedure (Yoder et al., 2014) was employed, modified, and programmed to operate using Python (Python Programming Language, v. 2.7) and SAS. A total of 2.761×106 records received from four commercial feed testing laboratories were used to develop the procedures and to construct tables summarizing feed composition. Briefly, feed names and variables across laboratories were standardized before the erroneous datapoints and duplicated samples were removed. Histogram, univariate, and principal component analyses were used to identify and remove outliers having key nutrients outside of the mean ± 3.5×SD. Clustering analyses were conducted to identify groups of feeds within a named feedstuff. Aside from the clustering step that was programmed in Python to automatically execute SAS, all steps were programmed and automatically conducted using Python followed by a manual evaluation of the resulting Pearson correlation matrixes and clusters. The input data contained 94, 162, 270, and 42 feeds, respectively, for laboratories 1 through 4 and were composed of 28 to 37 nutrients. The resulting database included 173 feeds (1.489×106records) with 111 feeds having more than 1 cluster. The developed procedures effectively classified byproducts (bakery byproducts, brewers grains, distillers grains and solubles, rice bran), forage (legume vs. grass, mature vs. immature and mid-maturity), and oilseeds vs. meal (cottonseed, canola seed, linseed/flaxseed, soybeans, sunflower) into distinct sub-populations. Results from these analyses provide a robust tool for the National Animal Nutrition Program (A National Research Support Project supported by USDA-NIFA and the State Agricultural Experiment Stations) to efficiently and consistently construct and update large feed datasets in an accurate, precise, and timely manner. This approach may also be used by commercial laboratories, feed manufacturers, animal producers, and other professionals to process feed composition datasets.
Keywords: automation, feed composition database, statistics